What is an NLP chatbot, and do you ACTUALLY need one? RST Software
The bot will send accurate, natural, answers based off your help center articles. Meaning businesses can start reaping the benefits of support automation in next to no time. With the rise of generative AI chatbots, we’ve now entered a new era of natural language processing.
And with the astronomical rise of generative AI — heralding a new era in the development of NLP — bots have become even more human-like. NLP allows computers and algorithms to understand human interactions via various languages. In order to process a large amount of natural language data, an AI will definitely need NLP or Natural Language Processing. Currently, we have a number of NLP research ongoing in order to improve the AI chatbots and help them understand the complicated nuances and undertones of human conversations.
Integrating & implementing an NLP chatbot
NLP is a subfield of AI that deals with the interaction between computers and humans using natural language. It is used in chatbot development to understand the context and sentiment of the user’s input and respond accordingly. While conversational AI chatbots can digest a users’ questions or comments and generate a human-like response, generative AI chatbots can take this a step further by generating new content as the output. This new content could look like high-quality text, images and sound based on LLMs they are trained on.
What Is A Chatbot? Everything You Need To Know – Forbes
What Is A Chatbot? Everything You Need To Know.
Posted: Mon, 26 Feb 2024 23:15:00 GMT [source]
Although hard to quantify initially, it is an important factor to consider in the long-term ROI calculations. Beyond transforming support, other types of repetitive tasks are ideal for integrating NLP chatbot in business operations. For example, if a user first asks about refund policies and then queries about product quality, the chatbot can combine these to provide a more comprehensive reply.
Understanding How NLP Works in Chatbots
NLP systems like translators, voice assistants, autocorrect, and chatbots attain this by comprehending a wide array of linguistic components such as context, semantics, and grammar. NLP and other machine learning technologies are making chatbots effective in doing the majority of conversations easily without human assistance. Primarily focused on machine reading comprehension, NLU gets the chatbot to comprehend what a body of text means.
By the end of this guide, beginners will have a solid understanding of NLP and chatbots and will be equipped with the knowledge and skills needed to build their chatbots. Whether one is a software developer looking to explore the world of NLP and chatbots or someone looking to gain a deeper understanding of the technology, this guide is an excellent starting point. Most top banks and insurance providers have already integrated chatbots into their systems and applications to help users with various activities. These bots for financial services can assist in checking account balances, getting information on financial products, assessing suitability for banking products, and ensuring round-the-clock help.
In fact, our case study shows that intelligent chatbots can decrease waiting times by up to 97%. This helps you keep your audience engaged and happy, which can boost your sales in the long run. On average, chatbots can solve about 70% of all your customer queries.
First we will create a function “utteranceToFeatures” than given a text (the utterance) will return the features object as the input of the example. The method chain is to build a pipeline of functions, and featuresToDict converts an array of features to the object format. Through this article you’ll learn theory, and later you’ll build your own NLP.
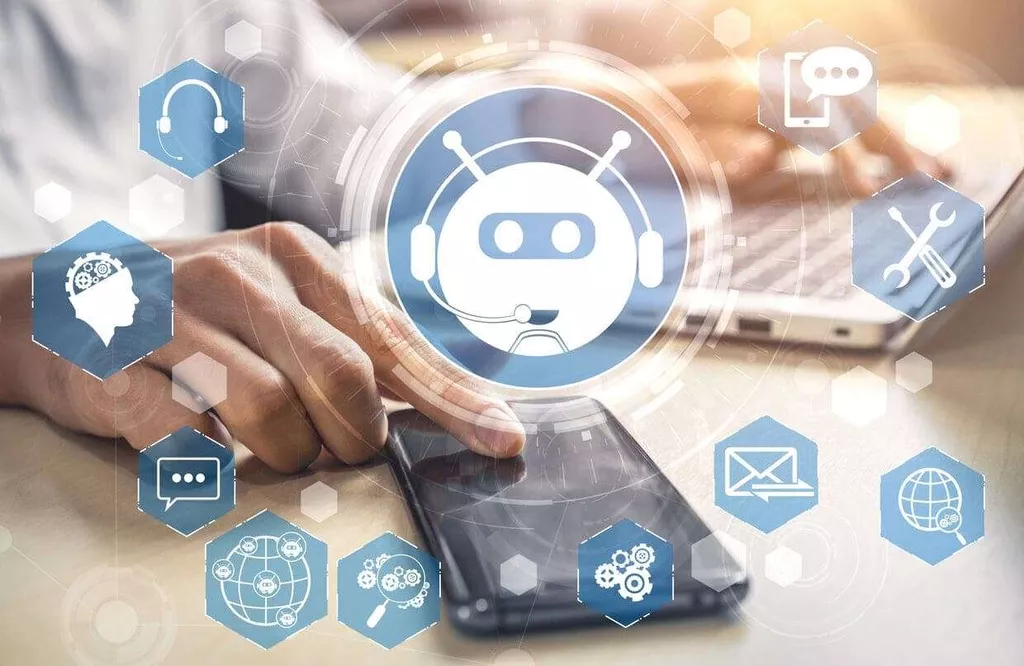
Artificial intelligence is a larger umbrella term that encompasses NLP and other AI initiatives like machine learning. Natural language processing (NLP) chatbots provide a better, more human experience for customers — unlike a robotic and impersonal experience that old-school answer bots are infamous for. You also benefit from more automation, zero contact resolution, better lead generation, and valuable feedback collection. To design the bot conversation flows and chatbot behavior, you’ll need to create a diagram.
Happy users and not-so-happy users will receive vastly varying comments depending on what they tell the chatbot. Chatbots may take longer to get sarcastic users the information that they need, because as we all know, sarcasm on the internet can sometimes be difficult to decipher. NLP powered chatbots require AI, or Artificial Intelligence, in order to function. These bots require a significantly greater amount of time and expertise to build a successful bot experience. Menu-based or button-based chatbots are the most basic kind of chatbot where users can interact with them by clicking on the button option from a scripted menu that best represents their needs. Depending on what the user clicks on, the simple chatbot may prompt another set of options for the user to choose until reaching the most suitable, specific option.
What is ChatGPT and why does it matter? Here’s what you need to know – ZDNet
What is ChatGPT and why does it matter? Here’s what you need to know.
Posted: Tue, 20 Feb 2024 08:00:00 GMT [source]
Natural language processing (NLP), in the simplest terms, refers to a behavioural technology that empowers AI to interact with humans using natural language. The aim is to read, decipher, understand, and analyse human languages to create valuable outcomes. It also means users don’t have to learn programming languages such as Python and Java to use a chatbot. This article explored five examples of chatbots that can talk like humans using NLP, including chatbots for language learning, customer service, personal finance, and news.
NLP_Flask_AI_ChatBot
As a cue, we give the chatbot the ability to recognize its name and use that as a marker to capture the following speech and respond to it accordingly. This is done to make sure that the chatbot doesn’t respond to everything that the humans are saying within its ‘hearing’ range. In simpler words, you wouldn’t want your chat bot nlp chatbot to always listen in and partake in every single conversation. Hence, we create a function that allows the chatbot to recognize its name and respond to any speech that follows after its name is called. Our chatbot pulls from many resource types to return highly matched answers to natural language queries.
If a user isn’t entirely sure what their problem is or what they’re looking for, a simple but likely won’t be up to the task. The benefits offered by NLP chatbots won’t just lead to better results for your customers. For example, one of the most widely used NLP chatbot development platforms is Google’s Dialogflow which connects to the Google Cloud Platform. If you really want to feel safe, if the user isn’t getting the answers he or she wants, you can set up a trigger for human agent takeover. Lack of a conversation ender can easily become an issue and you would be surprised how many NLB chatbots actually don’t have one. There are many who will argue that a chatbot not using AI and natural language isn’t even a chatbot but just a mare auto-response sequence on a messaging-like interface.
After the ai chatbot hears its name, it will formulate a response accordingly and say something back. Here, we will be using GTTS or Google Text to Speech library to save mp3 files on the file system which can be easily played back. And now the train function that given the neural network, the inputs (xs) and the classes (ys) will return the trained network. To develop the neural network we will use brain.js, that allows to develop classifiers in a simple way and with good enough performance. Tensorflow.js can be used but the code will be more complex for the same result.
Building upon the menu-based chatbot’s simple decision tree functionality, the rules-based chatbot employs conditional if/then logic to develop conversation automation flows. Train the chatbot to understand the user queries and answer them swiftly. The chatbot will engage the visitors in their natural language and help them find information about products/services. By helping the businesses build a brand by assisting them 24/7 and helping in customer retention in a big way. Visitors who get all the information at their fingertips with the help of chatbots will appreciate chatbot usefulness and helps the businesses in acquiring new customers.
Next, our AI needs to be able to respond to the audio signals that you gave to it. Now, it must process it and come up with suitable responses and be able to give output or response to the human speech interaction. This method ensures that the chatbot will be activated by speaking its name. You can foun additiona information about ai customer service and artificial intelligence and NLP. NLP or Natural Language Processing has a number of subfields as conversation and speech are tough for computers to interpret and respond to.
To make NLP work for particular goals, users will need to define all the types of Entities and Intents that the user wants the bot to recognise. In other words, users will create several NLP models, one for every Entity or Intent you need your chatbot to be able to identify. So, for example, you might build an NLP Intent model so that the bot can listen out for whether the user wishes to make a purchase.
Disney used NLP technology to create a chatbot based on a character from the popular 2016 movie, Zootopia. Users can actually converse with Officer Judy Hopps, who needs help solving a series of crimes. If you don’t want to write appropriate responses on your own, you can pick one of the available chatbot templates. When you first log in to Tidio, you’ll be asked to set up your account and customize the chat widget. The widget is what your users will interact with when they talk to your chatbot. You can choose from a variety of colors and styles to match your brand.
First, this kind of chatbot may take longer to understand the customers’ needs, especially if the user must go through several iterations of menu buttons before narrowing down to the final option. Second, if a user’s need is not included as a menu option, the chatbot will be useless since this chatbot doesn’t offer a free text input field. Although rule-based chatbots have limitations, they can effectively serve specific business functions. For example, they are frequently deployed in sectors like banking to answer common account-related questions, or in customer service for troubleshooting basic technical issues.
- Just remember that each Visitor Says node that begins the conversation flow of a bot should focus on one type of user intent.
- The benefits offered by NLP chatbots won’t just lead to better results for your customers.
- To increase the power of apps already in use, well-designed chatbots can be integrated into the software an organization is already using.
- A good NLP engine can make all the difference between a self-service chatbot that offers a great customer experience and one that frustrates your customers.
Improve customer service satisfaction and conversion rates by choosing a chatbot software that has key features. Take one of the most common natural language processing application examples — the prediction algorithm in your email. The software is not just guessing what you will want to say next but analyzes the likelihood of it based on tone and topic. Engineers are able to do this by giving the computer and “NLP training”. In essence, a chatbot developer creates NLP models that enable computers to decode and even mimic the way humans communicate.
Integrated into KLM’s Facebook profile, the chatbot handled tasks such as check-in notifications, delay updates, and distribution of boarding passes. Remarkably, within a short span, the chatbot was autonomously managing 10% of customer queries, thereby accelerating response times by 20%. Deploying a rule-based chatbot can only help in handling a portion of the user traffic and answering FAQs. NLP (i.e. NLU and NLG) on the other hand, can provide an understanding of what the customers “say”. Without NLP, a chatbot cannot meaningfully differentiate between responses like “Hello” and “Goodbye”.
The behavior of bots where AI is applied differs enormously from the behavior of bots where this is not applied. You can design, develop, and maintain chatbots using this powerful tool. IBM watsonx Assistant provides customers with fast, consistent and accurate answers across any application, device or channel. The terms chatbot, AI chatbot and virtual agent are often used interchangeably, which can cause confusion. While the technologies these terms refer to are closely related, subtle distinctions yield important differences in their respective capabilities.
You can come back to those when your bot is popular and the probability of that corner case taking place is more significant. Now it’s time to take a closer look at all the core elements that make NLP chatbot happen. Still, the decoding/understanding of the text is, in both cases, largely based on the same principle of classification.
This not only elevates the user experience but also gives businesses a tool to scale their customer service without exponentially increasing their costs. Natural Language Processing is a way for computer programs to converse with people in a language and format that people understand. With a user friendly, no-code/low-code platform you can build AI chatbots faster. When combined with automation capabilities like robotic process automation (RPA), users can accomplish tasks through the chatbot experience. Being deeply integrated with the business systems, the AI chatbot can pull information from multiple sources that contain customer order history and create a streamlined ordering process.
- Rasa is an open-source conversational AI framework that provides tools to developers for building, training, and deploying machine learning models for natural language understanding.
- Learn 4 steps to activate employees as brand ambassadors at only a fraction of paid advertising costs.
- Following the logic of classification, whenever the NLP algorithm classifies the intent and entities needed to fulfil it, the system (or bot) is able to “understand” and so provide an action or a quick response.
Then there’s an optional step of recognizing entities, and for LLM-powered bots the final stage is generation. These steps are how the chatbot to reads and understands each customer message, before formulating a response. To a human brain, all of this seems really simple as we have grown and developed in the presence of all of these speech modulations and rules. However, the process of training an AI chatbot is similar to a human trying to learn an entirely new language from scratch. The different meanings tagged with intonation, context, voice modulation, etc are difficult for a machine or algorithm to process and then respond to. NLP technologies are constantly evolving to create the best tech to help machines understand these differences and nuances better.
From categorizing text, gathering news and archiving individual pieces of text to analyzing content, it’s all possible with NLU. This includes making the chatbot available to the target audience and setting up the necessary infrastructure to support the chatbot. Before building a chatbot, it is important to understand the problem you are trying to solve.
The ultimate objective of NLP is to read, decipher, understand, and make sense of human language in a valuable way. Properly set up, a chatbot powered with NLP will provide fewer false positive outcomes. This is because NLP powered chatbots will properly understand customer intent to provide the correct answer to the customer query. You can harness the potential of the most powerful language models, such as ChatGPT, BERT, etc., and tailor them to your unique business application. Domain-specific chatbots will need to be trained on quality annotated data that relates to your specific use case.
In addition, conversational analytics can analyze and extract insights from natural language conversations, typically between customers interacting with businesses through chatbots and virtual assistants. Traditional text-based chatbots learn keyword questions and the answers related to them — this is great for simple queries. However, keyword-led chatbots can’t respond to questions they’re not programmed for. This limited scope leads to frustration when customers don’t receive the right information.
For bots without Natural Language Processing, a user has to go through a sequence of button and menu selections, without the option of text inputs. Tokenization is the process of dividing text into a set of meaningful pieces, such as words or letters, and these pieces are called tokens. This is an important step in building a chatbot as it ensures that the chatbot is able to recognize meaningful tokens.
A simple and powerful tool to design, build and maintain chatbots- Dashboard to view reports on chat metrics and receive an overview of conversations. It can identify spelling and grammatical errors and interpret the intended message despite the mistakes. This can have a profound impact on a chatbot’s ability to carry on a successful conversation with a user.
After these steps have been completed, we are finally ready to build our deep neural network model by calling ‘tflearn.DNN’ on our neural network. After the bag-of-words have been converted into numPy arrays, they are ready to be ingested by the model and the next step will be to start building the model that will be used as the basis for the chatbot. For our chatbot and use case, the bag-of-words will be used to help the model determine whether the words asked by the user are present in our dataset or not. So far, we’ve successfully pre-processed the data and have defined lists of intents, questions, and answers. In this guide, we’ll walk you through how you can use Labelbox to create and train a chatbot. For the particular use case below, we wanted to train our chatbot to identify and answer specific customer questions with the appropriate answer.
Investing in any technology requires a comprehensive evaluation to ascertain its fit and feasibility for your business. Here is a structured approach to decide if an NLP chatbot aligns with your organizational objectives. For example, if several customers are inquiring about a specific account error, the chatbot can proactively notify other users who might be impacted. ” the chatbot can understand this slang term and respond with relevant information. Users would get all the information without any hassle by just asking the chatbot in their natural language and chatbot interprets it perfectly with an accurate answer. This represents a new growing consumer base who are spending more time on the internet and are becoming adept at interacting with brands and businesses online frequently.
With REVE, you can build your own NLP chatbot and make your operations efficient and effective. They can assist with various tasks across marketing, sales, and support. To add more layers of information, you must employ various techniques while managing language. In getting started with NLP, it is vitally necessary to understand several language processing principles.
The next step will be to define the hidden layers of our neural network. The below code snippet allows us to add two fully connected hidden layers, each with 8 neurons. For this step, we’ll be using TFLearn and will start by resetting the default graph data to get rid of the previous graph settings. We recommend storing the pre-processed lists and/or numPy arrays into a pickle file so that you don’t have to run the pre-processing pipeline every time. To create a bag-of-words, simply append a 1 to an already existent list of 0s, where there are as many 0s as there are intents.
Recent Comments